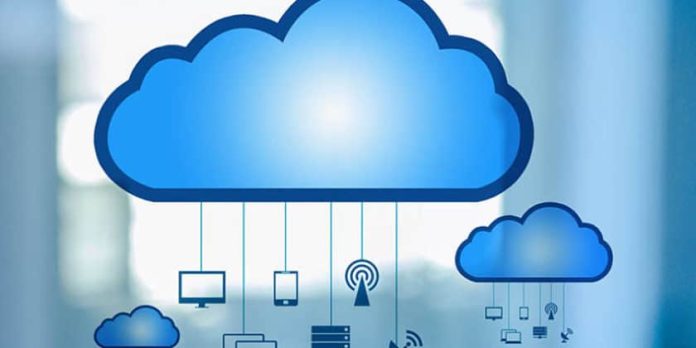
You probably heard the term “data lake” if you’re surrounded by the latest technology notions around crucial data. The picture has a vast water tank – that’s why it’s a data lake: a data tank solely.
There is no doubt that this information is the most valuable commodity for a business. But raising awareness, creating perspectives and turning them into decisions is even more important.
As data continues to grow in volume, data analytics pipelines need to be scalable to adjust the rate of change. And for this reason, choosing the better option in the cloud makes perfect sense (because the cloud provides scalability and flexibility on demand).
The data lake is defined here
In its basic state, a data lake contains a lot of raw and unstructured data.
All you need is a platform that allows you to access the data silos, so you can use a mainframe if you like. For processing, the data is transferred to other servers. Most companies use Snowflake, a platform that handles numerous data tasks on one platform, as it is designed to quickly process massive data sets and is employed in a huge data environment where the data lake may probably be used.
Data Lake Vs. data warehouse
Nothing new is the data warehouses; for decades data warehouses have existed. Although comparing them with data lakes is natural, it is some very specific differences that make both data warehouses and data lakes different from each other, from the kind of data kept to the manner in which they are handled.
The one main difference is that Data lakes do not require specialized hardware
Data lakes are more flexible
As we mentioned, a data lake contains a lot of raw, unstructured data in its natural format, and the data warehouse is structured far more in folders, lines and columns. A data lake is therefore far more flexible than a data store in terms of its data.
Before you do anything about it, you must actually understand the data as warehouse data is very structured. You can bring it from source data to structured projections iteratively via a maturity cycle while using a Data Lake. This can be seen along the route; we must not focus on data engineers and IT for the production of this data before it can be utilized.
Every data element in the data lake has a single identity and a wide range of metadata tags are included. When a business query is conducted on the basis of a specified metadata, all tagged data is evaluated.
Instead, data lakes do not contain a fundamental database unlike a data warehouse, instead these lakes use a flat file system. You must select data and columns with a database before you can write them. The compromise is that inserting the data in a database may take some time, but in a query it is faster than in a data lake that processes them as read.
So what do you think now? What kind of data source is best for your business? Still, confused? India Snowflake companies can help you access and use all the data you required for your business.